Improving Audio Machine Learning Infrastructure at Ubenwa
Learn how Ubenwa, a growing force in sound-based infant medical diagnostics, 2x efficiency & improved scalability with streamable, standardized Deep Lake datasets
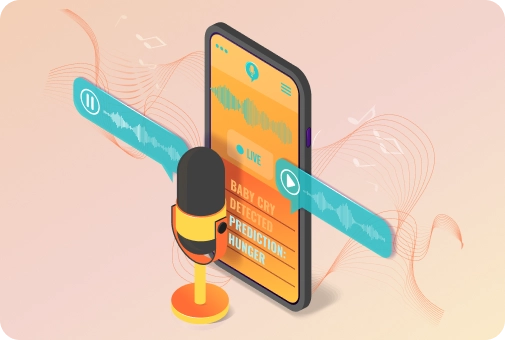
Processing
Company Background
Ubenwa develops AI-powered software for the early detection of neurological and respiratory conditions in infants using their cry. You've probably wondered at least once - why is my baby crying? Ubenwa is addressing just that. The company has a machine learning organization with 3 machine learning researchers (and some occasional interns!). The startup is in the early stages of developing a machine learning system that can accurately predict neonatal distress, a critical need, especially in developing countries. The company faced several challenges in building a scalable and efficient data infrastructure to support its machine learning models. Upon joining the company, our interviewee, Arsenii, was tasked with solving these challenges
Meet the Interviewee
Arsenii is a Lead Machine Learning Engineer at Ubenwa who has been with the company for over six months. Arsenii was also responsible for building the data infrastructure and ensuring the efficient operation of the machine learning models. Before Ubenwa, Arsenii experienced all the bottlenecks of building complex data infrastructure in a quickly growing startup. He evaluated several plug-and-play solutions and chose Activeloop thanks to the quick time-to-value he experienced with Deep Lake.
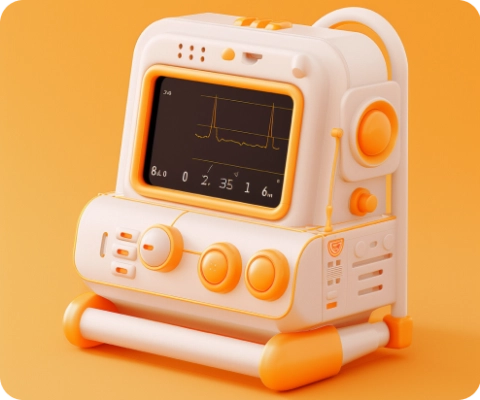
“Accessing data in the cloud is like walking through quicksand, and relying on slow and unreliable file systems is like sinking deeper. Downloading data every time you run an experiment is like carrying a heavy burden that slows you down, and eventually, it might break you (and the training process). Deep Lake's on-the-fly streaming was an excellent choice for us: it was really easy to set up, and it started to bring the value of fast data loading from day one.”
Arsenii Gorin
Lead Machine Learning Engineer at Ubenwa
The Challenges
Before Activeloop, Ubenwa ML team faced several challenges in building a scalable and efficient data infrastructure.
- 1
Lack of Standardization
The data infrastructure was in its early stages, and there was no standardization in how data was loaded or processed. This led to a fragmented and disorganized data pipeline, making it difficult to scale the system.
- 2
Inefficient Data Loading
Ubenwa ML team spent a lot of time on the data loading process, which was not optimized for the company's use case. This resulted in slow and inefficient machine learning training pipelines. More importantly, for PyTorch training in the cloud, for instance, one could spend a lot of time loading data only after it catches an error in the training code.
- 3
No Support for Audio Data
Ubenwa's primary data source was audio recordings of crying babies, which the existing data infrastructure was not optimized for. This was a significant bottleneck in the system, as audio data is critical for building accurate machine learning models.
Solution
Speed, data quality, single source of truth, & easy-to-use UI. Activeloop was the solution that Arsenii was looking for to solve the problems faced at Ubenwa. Activeloop is a scalable and efficient data infrastructure platform that supports audio data and provides a standard way of processing and loading data.
Results
2x the efficiency, standardization of ML datasets quality, plug-and-play scalable audio infrastructure for machine learning. Activeloop significantly improved the data infrastructure at Ubenwa, improving the efficiency and scalability of the system. Some of the key results were
Increased Efficiency by 2x
The Data Loading Process was Optimized, Reducing the Time Spent on Data Loading - From Two Weeks to Just One Week.Standardization of Datasets for Machine Learning
Activeloop Provided a Standard Way of Processing and Loading Data, Resulting in a More Organized and Streamlined Data Pipeline.Support for Audio Data
Activeloop Supported Audio Data, a Critical Requirement for Ubenwa's Machine Learning Models. This Allowed the Ubenwa ML Team to Efficiently Process Audio Recordings of Neonatal Distress, Which Was Impossible Before.Improved Scalability
The Efficient and Standardized Data Pipeline Enabled Ubenwa to Scale its Machine Learning Models More Efficiently, Resulting in a More Scalable System.
Concluding Remarks
Critical solution for scaling startups. Activeloop was a critical solution for Ubenwa's data infrastructure, providing a scalable and efficient platform for processing and loading data. The optimized data pipeline and support for audio data significantly improved the efficiency and scalability of Ubenwa's machine learning models. By adopting Activeloop, Ubenwa was able to build a more efficient and scalable system, accelerating towards their goal of detecting neonatal distress more accurately.
How Bayer Radiology Uses Database for AI to Disrupt Healthcare with GenAI
Learn how Bayer Radiology, a division of a pharmaceutical powerhouse, used a secure, efficient, & scalable database for AI to pioneer medical GenAI workflows
Read more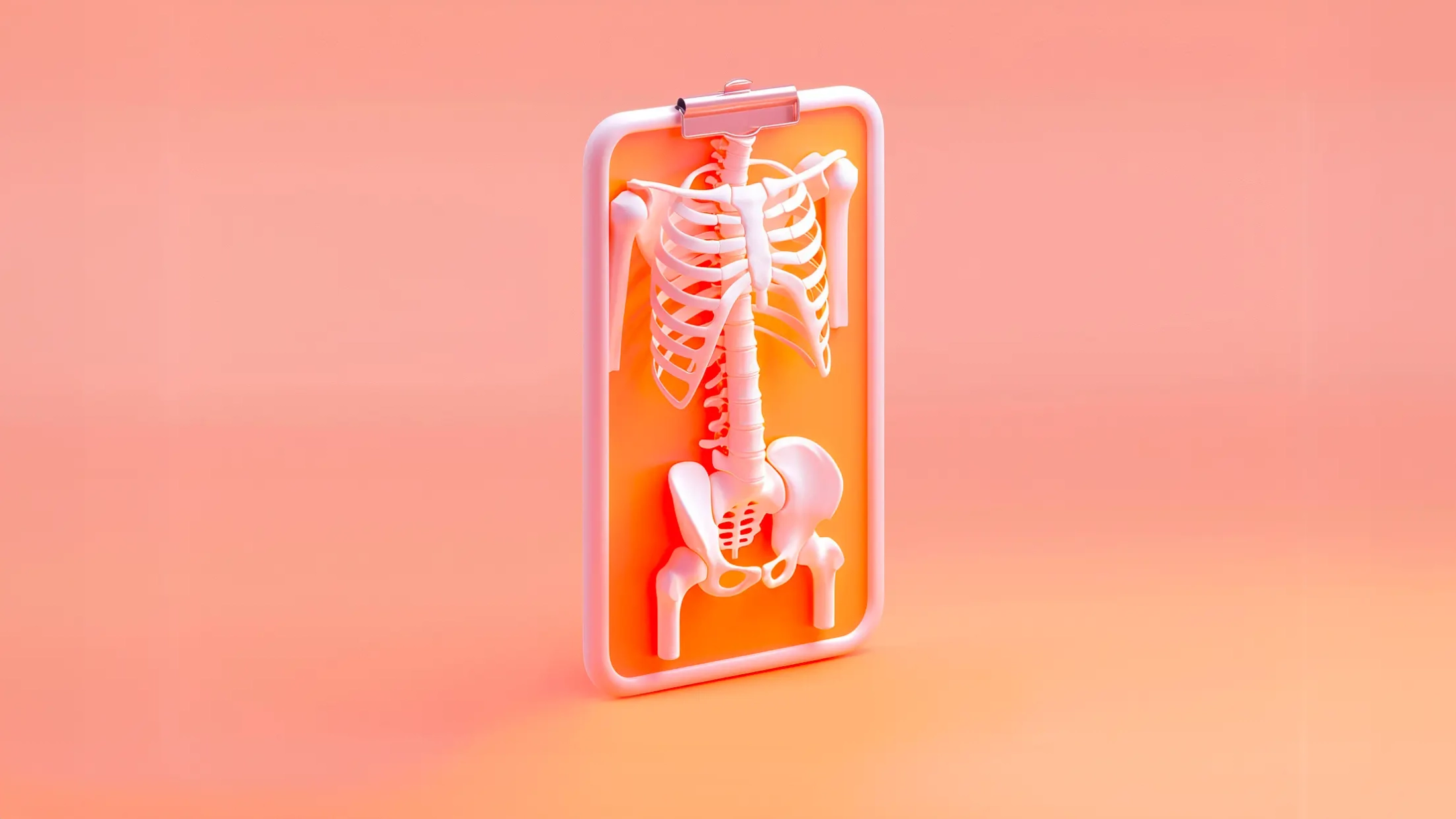
Increase in Lawyer Productivity with Hercules.ai by 18.5%
Discover how Ropers Majeski, a leading law firm, utilized Hercules.AI, powered by Activeloop's cutting-edge enterprise data solutions, to achieve remarkable productivity gains and cost efficiencies with LLMs
Read more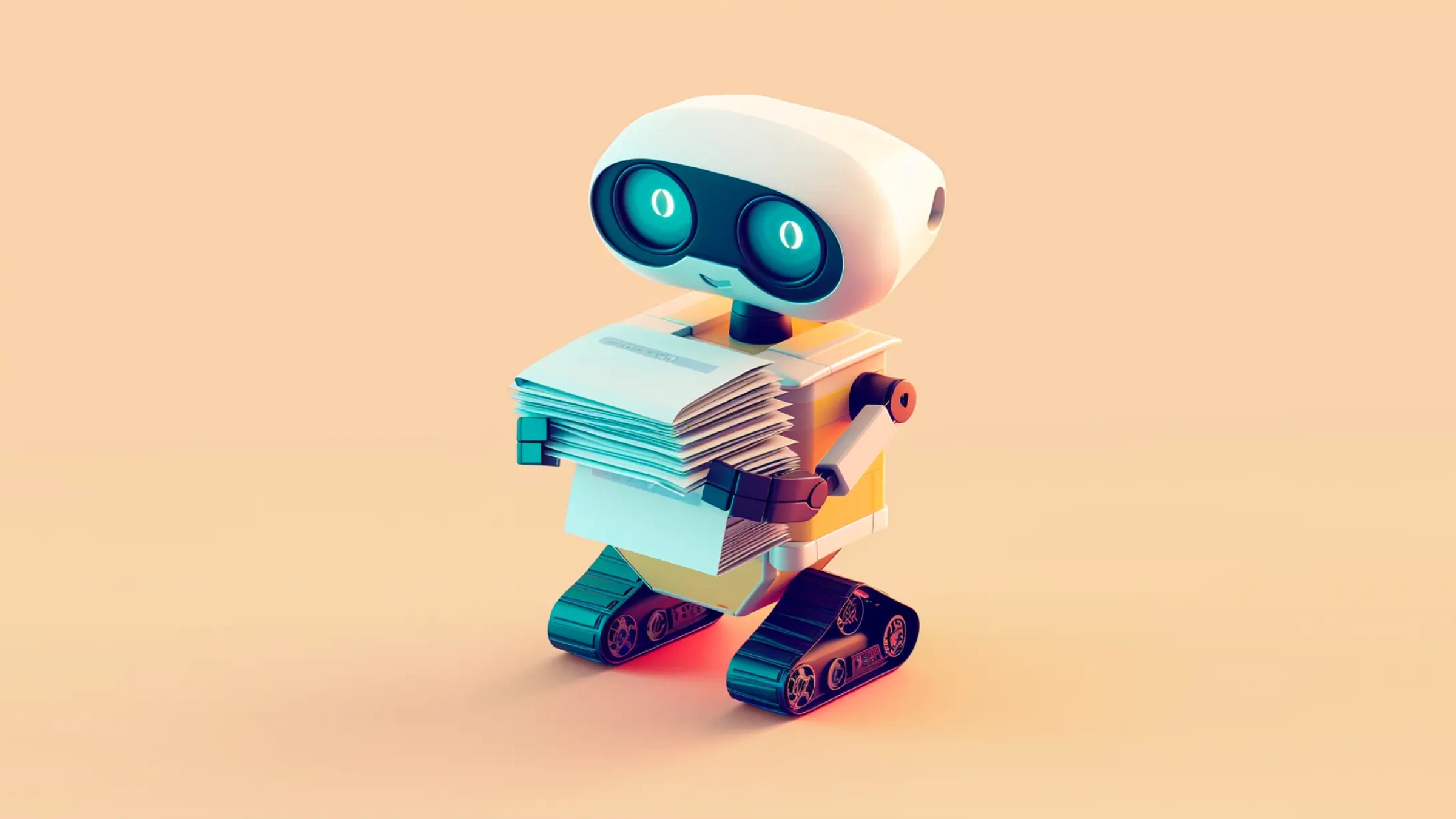